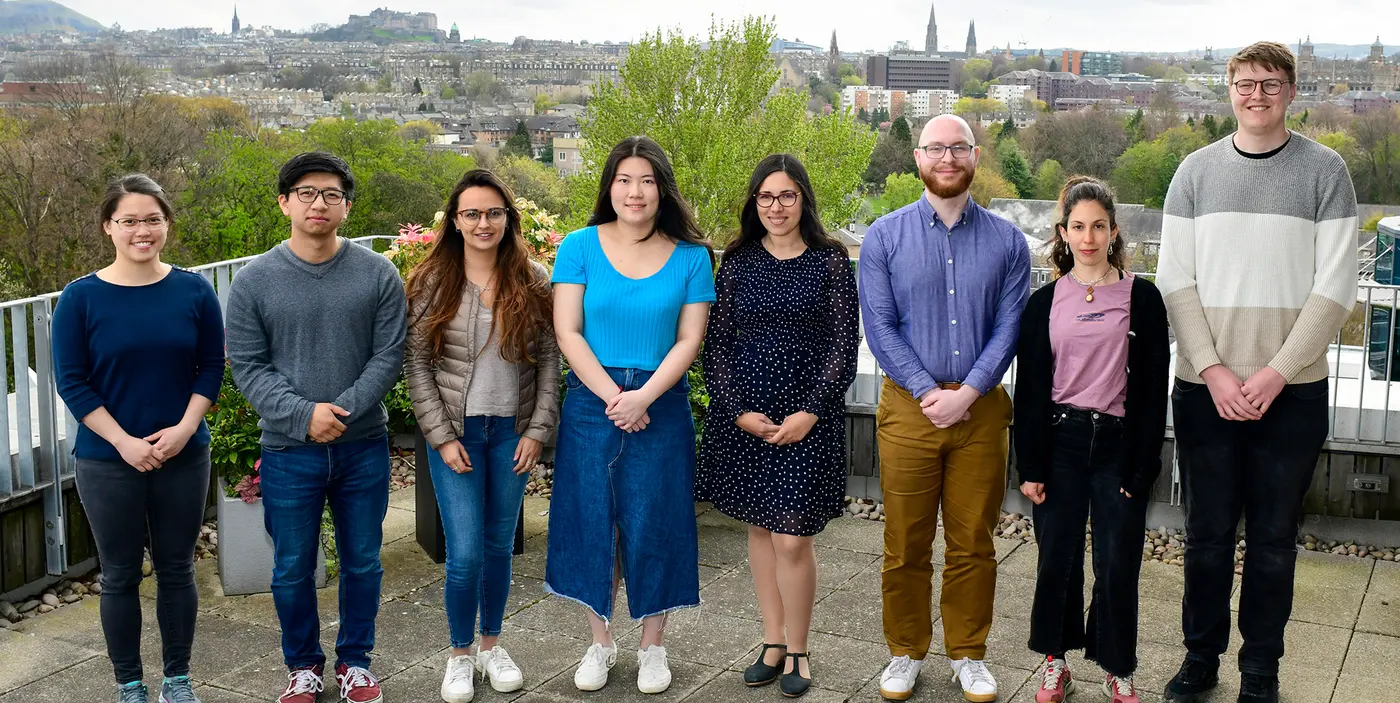
Vallejos Group
Understanding heterogeneity in complex biomedical data
About
While biomedical data sometimes classifies as “big data” (where the number of samples and/or variables is large), complexity is its most prominent feature. This arises from a combination of different sources of heterogeneity: heterogeneity across individuals in a population (e.g. response to treatment), heterogeneity in terms of the type of data we collect (e.g. health records & genomics) and heterogeneity that is introduced by the data collection process (e.g. measurement error).
We focus on the development of novel statistical methodology to address and study these sources of heterogeneity. This is a highly multidisciplinary task: from the understanding of complex biomedical problems and technologies, to the development of new methodology and the implementation of open-source analysis tools. Our current research focuses on two areas of application. Firstly, single-cell RNA-sequencing, a cutting-edge experimental technique that allows genome-wide quantification of gene expression on a cell-by-cell basis. Secondly, electronic health records research, to develop predictive models based on observational data that is routinely collected by health providers (e.g. NHS). Developing computational tools that can make full advantage of the rich information provided by these data sources is ought to improve our understanding of health and disease, playing an important role in precision medicine initiatives.
News
Jan 31, 2024 | Nathan has passed his viva! |
---|---|
May 26, 2022 | Cata got tenure after a successful ESAT review. |
May 6, 2022 | Alan has passed his viva! |
Oct 1, 2021 | Linda and Elena have joined the group! |
Selected Publications
- medRxivLarge-scale clustering of longitudinal faecal calprotectin and C-reactive protein profiles in inflammatory bowel diseasemedRxiv Jan 2025
Background Crohn’s disease (CD) and ulcerative colitis (UC) are highly heterogeneous, dynamic and unpredictable, with a marked disconnect between symptoms and intestinal inflammation. Attempts to classify inflammatory bowel disease (IBD) subphenotypes to inform clinical decision making have been limited. We aimed to describe the latent disease heterogeneity by modelling routinely collected faecal calprotectin (FC) and CRP data, describing dynamic longitudinal inflammatory patterns in IBD.Methods In this longitudinal study, we analysed patient-level post-diagnosis measurements of FC and CRP in two European cohorts. Latent class mixed models were used to cluster individuals with similar longitudinal profiles. Associations between cluster assignment and baseline characteristics were quantified using multinomial logistic regression. Differences in advanced therapy use across clusters were also explored. Finally, we considered uncertainty in cluster assignments with respect to follow-up length and the overlap between FC and CRP clusters. We included 1036 patients in the FC discovery analysis (Lothian) with a total of 10545 FC observations (median 9 per subject, IQR 6–13), and 7880 patients in the replication (Denmark). The CRP discovery analysis consisted of 1838 patients with 49364 measurements (median 20 per subject; IQR 10–36), with 10041 patients in the replication cohort.Findings Eight distinct clusters of inflammatory behaviour over time were identified in the FC and CRP analysis for the Scottish cohort. This model was then applied to the Danish replication cohort, with similar patterns observed in both the Scottish and Danish populations. The clusters, FC1–8 and CRP1–8, were ordered from the lowest cumulative inflammatory burden to the highest. The clusters included groups with high diagnostic levels of inflammation which rapidly normalised, groups where high inflammation levels persisted throughout the full seven years of observation, and a series of intermediates including delayed remitters and relapsing remitters. CD and UC patients were unevenly distributed across the clusters. In UC, male sex was associated with the poorest prognostic cluster (FC8). The use and timing of advanced therapy was associated with cluster assignment, with the highest use of early advanced therapy in FC1. Of note, FC8 and CRP8 captured consistently high patterns of inflammation despite a high proportion of patients receiving advanced therapy, particularly for CD individuals. We observed that uncertainty in cluster assignments was higher for individuals with short longitudinal follow-up, particularly between clusters capturing similar earlier inflammation patterns. There was broadly poor agreement between FC and CRP clusters in keeping with the need to monitor both in clinical practice.Interpretation Distinct patterns of inflammatory behaviour over time are evident in patients with IBD. Cluster assignment is associated with disease type and both the use and timing of advanced therapy. These data pave the way for a deeper understanding of disease heterogeneity in IBD and enhanced patient stratification in the clinic.Competing Interest StatementNP has served as a speaker for Janssen, Takeda and Pfizer. BG has acted as consultant to Galapagos and Abbvie and as speaker for Abbvie, Jansen, Takeda, Pfizer and Galapagos. GRJ has served as a speaker for Takeda, Janssen, Abbvie, Fresnius and Ferring. TJ has served as a speaker and/or consultant for Ferring and Pfizer. CWL has acted as a speaker and/or consultant to AbbVie, Janssen, Takeda, Pfizer, Galapagos, GSK, Gilead, Vifor Pharma, Ferring, Dr Falk, BMS, Boehringer Ingelheim, Eli Lilly, Merck, Novartis, Sandoz, Celltrion, Cellgene, Amgen, Samsung Bioepis, Fresenius Kabi, Tillotts, Kuma Health, Trellus Health and Iterative Health. None of the other authors report any conflicts of interest.Funding StatementCWL is funded by a UKRI (UK Research and Innovation) Future Leaders Fellowship ’Predicting outcomes in IBD’ (MR/S034919/1). G-RJ is funded by a Wellcome Trust Clinical Research Career Development Fellowship. NC-C was partially supported by the Medical Research Council and The University of Edinburgh via a Precision Medicine PhD studentship (MR/N013166/1). MVV, AS, and TJ are funded by a Center of Excellence Grant (DNRF148) to TJ from the Danish National Research Foundation.Author DeclarationsI confirm all relevant ethical guidelines have been followed, and any necessary IRB and/or ethics committee approvals have been obtained.YesThe details of the IRB/oversight body that provided approval or exemption for the research described are given below:Usage of the Scottish dataset was approved by the local Caldicott Guardian (Project ID: CRD18002, registered NHS Lothian information asset #IAR-954). In Denmark, studies based on registry data alone are not required to obtain permission from the regional ethics committees as confirmed by The Central Denmark Region Committees on Health Research Ethics (legislation: 1-10-72-148-19)I confirm that all necessary patient/participant consent has been obtained and the appropriate institutional forms have been archived, and that any patient/participant/sample identifiers included were not known to anyone (e.g., hospital staff, patients or participants themselves) outside the research group so cannot be used to identify individuals.YesI understand that all clinical trials and any other prospective interventional studies must be registered with an ICMJE-approved registry, such as ClinicalTrials.gov. I confirm that any such study reported in the manuscript has been registered and the trial registration ID is provided (note: if posting a prospective study registered retrospectively, please provide a statement in the trial ID field explaining why the study was not registered in advance).YesI have followed all appropriate research reporting guidelines, such as any relevant EQUATOR Network research reporting checklist(s) and other pertinent material, if applicable.YesAs the data collected for this study has been derived from unconsented patient data, it is not possible to share subject-level data with external entities. Detailed summary level data is available online at https://vallejosgroup.github.io/Lothian-IBDR. The code used to conduct the analysis is also publicly available (https://github.com/VallejosGroup/Lothian-IBDR). https://vallejosgroup.github.io/IBD-Inflammatory-Patterns/ https://github.com/VallejosGroup/IBD-Inflammatory-Patterns
@article{Constantine-Cooke2024.11.08.24316916, abbr = {medRxiv}, bibtex_show = {true}, selected = {true}, author = {Constantine-Cooke, Nathan and Vestergaard, Marie Vibeke and Plevris, Nikolas and Monterrubio-G{\'o}mez, Karla and Ramos Belinch{\'o}n, Clara and Ong, Solomon and Elford, Alexander T. and Gros, Beatriz and Sazonovs, Aleksejs and Jones, Gareth-Rhys and Jess, Tine and Vallejos, Catalina A. and Lees, Charlie W.}, title = {Large-scale clustering of longitudinal faecal calprotectin and C-reactive protein profiles in inflammatory bowel disease}, elocation-id = {2024.11.08.24316916}, year = {2025}, month = jan, doi = {10.1101/2024.11.08.24316916}, publisher = {Cold Spring Harbor Laboratory Press}, url = {https://www.medrxiv.org/content/early/2025/01/17/2024.11.08.24316916}, eprint = {https://www.medrxiv.org/content/early/2025/01/17/2024.11.08.24316916.full.pdf}, journal = {medRxiv} }
- Clin EpigenBlood-based epigenome-wide association study and prediction of alcohol consumptionClinical Epigenetics Jan 2025
Alcohol consumption is an important risk factor for multiple diseases. It is typically assessed via self-report, which is open to measurement error through recall bias. Instead, molecular data such as blood-based DNA methylation (DNAm) could be used to derive a more objective measure of alcohol consumption by incorporating information from cytosine-phosphate-guanine (CpG) sites known to be linked to the trait. Here, we explore the epigenetic architecture of self-reported weekly units of alcohol consumption in the Generation Scotland study. We first create a blood-based epigenetic score (EpiScore) of alcohol consumption using elastic net penalized linear regression. We explore the effect of pre-filtering for CpG features ahead of elastic net, as well as differential patterns by sex and by units consumed in the last week relative to an average week. The final EpiScore was trained on 16,717 individuals and tested in four external cohorts: the Lothian Birth Cohorts (LBC) of 1921 and 1936, the Sister Study, and the Avon Longitudinal Study of Parents and Children (total N across studies > 10,000). The maximum Pearson correlation between the EpiScore and self-reported alcohol consumption within cohort ranged from 0.41 to 0.53. In LBC1936, higher EpiScore levels had significant associations with poorer global brain imaging metrics, whereas self-reported alcohol consumption did not. Finally, we identified two novel CpG loci via a Bayesian penalized regression epigenome-wide association study of alcohol consumption. Together, these findings show how DNAm can objectively characterize patterns of alcohol consumption that associate with brain health, unlike self-reported estimates.
@article{Bernabeu_2025, abbr = {Clin Epigen}, bibtex_show = {true}, selected = {true}, title = {Blood-based epigenome-wide association study and prediction of alcohol consumption}, volume = {17}, issn = {1868-7083}, url = {http://dx.doi.org/10.1186/s13148-025-01818-y}, doi = {10.1186/s13148-025-01818-y}, number = {1}, journal = {Clinical Epigenetics}, publisher = {Springer Science and Business Media LLC}, author = {Bernabeu, Elena and Chybowska, Aleksandra D. and Kresovich, Jacob K. and Suderman, Matthew and McCartney, Daniel L. and Hillary, Robert F. and Corley, Janie and Valdés-Hernández, Maria Del C. and Maniega, Susana Muñoz and Bastin, Mark E. and Wardlaw, Joanna M. and Xu, Zongli and Sandler, Dale P. and Campbell, Archie and Harris, Sarah E. and McIntosh, Andrew M. and Taylor, Jack A. and Yousefi, Paul and Cox, Simon R. and Evans, Kathryn L. and Robinson, Matthew R. and Vallejos, Catalina A. and Marioni, Riccardo E.}, year = {2025}, month = jan }
- NPJ DMDevelopment and assessment of a machine learning tool for predicting emergency admission in Scotlandnpj Digital Medicine Oct 2024
Emergency admissions (EA), where a patient requires urgent in-hospital care, are a major challenge for healthcare systems. The development of risk prediction models can partly alleviate this problem by supporting primary care interventions and public health planning. Here, we introduce SPARRAv4, a predictive score for EA risk that will be deployed nationwide in Scotland. SPARRAv4 was derived using supervised and unsupervised machine-learning methods applied to routinely collected electronic health records from approximately 4.8M Scottish residents (2013-18). We demonstrate improvements in discrimination and calibration with respect to previous scores deployed in Scotland, as well as stability over a 3-year timeframe. Our analysis also provides insights about the epidemiology of EA risk in Scotland, by studying predictive performance across different population sub-groups and reasons for admission, as well as by quantifying the effect of individual input features. Finally, we discuss broader challenges including reproducibility and how to safely update risk prediction models that are already deployed at population level.
@article{Liley_2024, abbr = {NPJ DM}, bibtex_show = {true}, selected = {true}, title = {Development and assessment of a machine learning tool for predicting emergency admission in Scotland}, volume = {7}, issn = {2398-6352}, url = {http://dx.doi.org/10.1038/s41746-024-01250-1}, doi = {10.1038/s41746-024-01250-1}, number = {1}, journal = {npj Digital Medicine}, publisher = {Springer Science and Business Media LLC}, author = {Liley, James and Bohner, Gergo and Emerson, Samuel R. and Mateen, Bilal A. and Borland, Katie and Carr, David and Heald, Scott and Oduro, Samuel D. and Ireland, Jill and Moffat, Keith and Porteous, Rachel and Riddell, Stephen and Rogers, Simon and Thoma, Ioanna and Cunningham, Nathan and Holmes, Chris and Payne, Katrina and Vollmer, Sebastian J. and Vallejos, Catalina A. and Aslett, Louis J. M.}, year = {2024}, month = oct }
- AI & EthicsEthical considerations of use of hold-out sets in clinical prediction model managementAI and Ethics Sep 2024
Clinical prediction models are statistical or machine learning models used to quantify the risk of a certain health outcome using patient data. These can then inform potential interventions on patients, causing an effect called performative prediction: predictions inform interventions which influence the outcome they were trying to predict, leading to a potential underestimation of risk in some patients if a model is updated on this data. One suggested resolution to this is the use of hold-out sets, in which a set of patients do not receive model derived risk scores, such that a model can be safely retrained. We present an overview of clinical and research ethics regarding potential implementation of hold-out sets for clinical prediction models in health settings. We focus on the ethical principles of beneficence, non-maleficence, autonomy and justice. We also discuss informed consent, clinical equipoise, and truth-telling. We present illustrative cases of potential hold-out set implementations and discuss statistical issues arising from different hold-out set sampling methods. We also discuss differences between hold-out sets and randomised control trials, in terms of ethics and statistical issues. Finally, we give practical recommendations for researchers interested in the use hold-out sets for clinical prediction models.
@article{Chislett_2024, abbr = {AI & Ethics}, bibtex_show = {true}, selected = {true}, title = {Ethical considerations of use of hold-out sets in clinical prediction model management}, issn = {2730-5961}, url = {http://dx.doi.org/10.1007/s43681-024-00561-z}, doi = {10.1007/s43681-024-00561-z}, journal = {AI and Ethics}, publisher = {Springer Science and Business Media LLC}, author = {Chislett, Louis and Aslett, Louis J. M. and Davies, Alisha R. and Vallejos, Catalina A. and Liley, James}, year = {2024}, month = sep }
- PLOS DHDifferential behaviour of a risk score for emergency hospital admission by demographics in Scotland—A retrospective studyPLOS Digital Health Dec 2024
@article{Thoma_2024, abbr = {PLOS DH}, bibtex_show = {true}, selected = {true}, title = {Differential behaviour of a risk score for emergency hospital admission by demographics in Scotland—A retrospective study}, volume = {3}, issn = {2767-3170}, url = {http://dx.doi.org/10.1371/journal.pdig.0000675}, doi = {10.1371/journal.pdig.0000675}, number = {12}, journal = {PLOS Digital Health}, publisher = {Public Library of Science (PLoS)}, author = {Thoma, Ioanna and Rogers, Simon and Ireland, Jillian and Porteous, Rachel and Borland, Katie and Vallejos, Catalina A. and Aslett, Louis J. M. and Liley, James}, editor = {Kuo, Po-Chih}, year = {2024}, month = dec, pages = {e0000675}, abtract = {The Scottish Patients at Risk of Re-Admission and Admission (SPARRA) score predicts individual risk of emergency hospital admission for approximately 80% of the Scottish population. It was developed using routinely collected electronic health records, and is used by primary care practitioners to inform anticipatory care, particularly for individuals with high healthcare needs. We comprehensively assess the SPARRA score across population subgroups defined by age, sex, ethnicity, socioeconomic deprivation, and geographic location. For these subgroups, we consider differences in overall performance, score distribution, and false positive and negative rates, using causal methods to identify effects mediated through age, sex, and deprivation. We show that the score is well-calibrated across subgroups, but that rates of false positives and negatives vary widely, mediated by various causes including variability in demographic characteristics, admission reasons, and potentially differential data availability. Our work assists practitioners in the application and interpretation of the SPARRA score in population subgroups.} }
- BiometA review on statistical and machine learning competing risks methodsBiometrical Journal Feb 2024
Abstract When modeling competing risks (CR) survival data, several techniques have been proposed in both the statistical and machine learning literature. State-of-the-art methods have extended classical approaches with more flexible assumptions that can improve predictive performance, allow high-dimensional data and missing values, among others. Despite this, modern approaches have not been widely employed in applied settings. This article aims to aid the uptake of such methods by providing a condensed compendium of CR survival methods with a unified notation and interpretation across approaches. We highlight available software and, when possible, demonstrate their usage via reproducible R vignettes. Moreover, we discuss two major concerns that can affect benchmark studies in this context: the choice of performance metrics and reproducibility.
@article{https://doi.org/10.1002/bimj.202300060, abbr = {Biomet}, bibtex_show = {true}, author = {Monterrubio-Gómez, Karla and Constantine-Cooke, Nathan and Vallejos, Catalina A.}, title = {A review on statistical and machine learning competing risks methods}, journal = {Biometrical Journal}, volume = {66}, number = {2}, pages = {2300060}, keywords = {competing risks, risk prediction, survival analysis, time-to-event data}, doi = {https://doi.org/10.1002/bimj.202300060}, url = {https://onlinelibrary.wiley.com/doi/abs/10.1002/bimj.202300060}, eprint = {https://onlinelibrary.wiley.com/doi/pdf/10.1002/bimj.202300060}, year = {2024}, month = feb, selected = {true} }